Welcome to part three of our blog series on the challenges of sanctions screening, which seeks to highlight the issues with and solutions to common, costly pitfalls in screening processes.
In last week’s blog we covered the expectation on businesses to be continually informed of and mitigate their sanction risks, and we outlined the penalties organisations face when this requirement is not observed.
In this blog, we delve into the biggest challenges in sanctions screening, with particular attention to issues with data, the causes of false positives, and how costly false positives and negatives can be for organisations.
Typical challenges in sanctions screening
The process of screening customers, suppliers, employees, and transactions is fraught with challenges which are driven by problems within data such as:
- Identity similarities
- Spelling mistakes and typos
- Data capture mistakes
- Poor data collection and organisation
- External data inconsistencies in format, datasets, and geographical coverage, making direct and reliable comparisons challenging
- Poor and insufficient data
Inadequate screening systems and technology
But the use of inadequate or legacy screening systems and technology is also a key factor. These are often slow at performing matches, inflexible and difficult to configure and require greater manual intervention, increasing the risk of error.
Criminal efforts to purposely undermine and derail the effectiveness of the screening process, such as false identities and frequent changes of address also contribute to the challenges in screening.
However, despite these challenges, organizations must continue to mitigate the risk of breaching regulations and meet these obligations.
What are the root causes of false positives in screening?
False positives are names, entities, or transactions that are incorrectly flagged as alerts or matches by the screening system.
The key root causes of false positives are:
- Inefficient matching strategy
- Inflexible screening process, which does not take the context of a match into account.
- Legacy screening systems using inadequate algorithms, leading to inflated levels of false positives.
- Legacy screening systems that require a long time to run and do not allow for the use of more sophisticated techniques in the screening process.
- Opaque box algorithms that require thresholds to be set carefully with thorough testing to avoid false negatives but can instead often generate false positives and miss some true hits.
- Legacy screening solutions that lack the ability to enhance matching results by leveraging additional data such as pictures, passport number or date of birth.
The problem with legacy screening systems
Legacy screening systems are outdated in terms of technology, standards, and processes. For example, systems solely built on relational databases do not lend themselves well to the performance and scalability requirements that a modern screening solution requires.
Here we compare how some of the most common sanctions screening system features vary between legacy and modern systems.

The impact of legacy screening systems and processes
The true cost of legacy sanctions screening systems and processes is hidden in the unnecessarily high levels of false positives and false negatives.
1) High levels of costly false positives
False positives are false matches, which must be dealt with by teams of analysts, performing the same checks repeatedly. The average false positives rate can reach up to 5-8% in legacy systems.
This means that if one million entities are screened, 50,000 to 80,000 will need to be manually reviewed. What is more, with an average number of 5-8 hits per entity, for every million entities there can be up to 400,000 hits to manually review.
If each false positive must be documented with an explanation as why it is a false positive, and that it takes between 30 seconds and one minute to review, the cost of reviewing false positives per million customers or transactions screened could exceed £200,000 (the cost of running a compliance team for the time needed to review false positives).

2) High levels of costly false negatives
False negatives can lead to sanctions violation and fines. In the US, the average sanctions breach fine between 2018 and 2020 was $20.4m. The enormity of this average is due to substantial fines levied upon UniCredit Bank and Standard Chartered Bank in 2019.
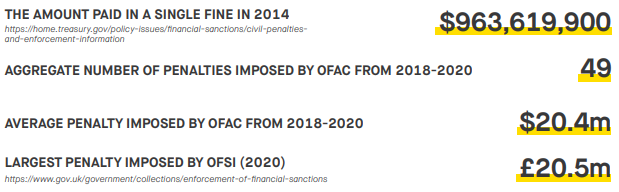
Sanctions screening: how to reduce false positives in client and transaction screening
Today’s blog has looked at the causes of false positives in sanctions screening processes, focusing on legacy systems and processes, and the costs of these problems for organisations if not addressed.
Over the next few chapters of this blog series, we will further discuss the challenges organisations face regarding sanctions risk management, introduce solutions, and talk about how the latest technology, like that of Napier, can minimise error in the screening process and protect from costly penalties.
This article is the third in a series of a larger paper authored by Napier.
If you would like to read the full paper, you can download it here.