At their very simplest, effective anti-money laundering frameworks must do two things - prevent would-be money launderers from entering the financial system in the first place and, if they do fall through the cracks, spot suspicious activity and report it to the relevant Financial Intelligence Unit.
But, the high levels of AML related regulatory fines and the tiny proportion of illicit funds that are recovered - despite the amount spent on financial crime compliance - show that the current methods for detecting financial crime are falling short.
Why is this the case?
One clear reason is that common approaches to transaction monitoring do not take customer behaviour into account, making it impossible to distinguish between what might be legitimate activity and what is criminality.
First - the data.
Most data that financial institutions hold on their customers - whether from transactions or from Know Your Customer (KYC), actually consists of a fairly limited number of attributes. If you look at files containing payment transaction details (from SWIFT or CHIPS or Fedwire), the data set consists solely of customer and beneficiary account details, the amount of the transfer, the currency and the date - and in some instances, even this may not be complete or accurate.
What there definitely won’t be is any contextual information about that transaction, the customer or the beneficiary.
Second - the monitoring methods.
For transaction monitoring, the majority of financial institutions are still using systems that create structured rules derived from red flags or indicators for a whole variety of financial crime typologies. I estimate that there are about 1100 red flags issued by bodies such as the Financial Action Task Force (FATF), Federal Financial Institutions Examinations Council (FFIEC), FINRA (Financial Institutions Regulatory Authority) and the Wolfsberg Group.
Only using rules for detecting suspicious transactions is problematic - and this is because rules do not take behaviour into account.
Why is customer behaviour so important to AML?
Successful transaction monitoring must be able to distinguish between behaviour that is in some way ‘normal’ and that which is indicative of criminal activity. But the definition of what is seen as ‘normal’ is fluid - and can change based on circumstances.
A couple of examples may help to illustrate this point.
In the US, the last hour of trading on the New York Stock Exchange on the third Friday in March, June, September and December is known as the ‘triple witching hour’ - the quarterly expiration date of stock market index futures and options and stock options. During this hour, there is a spike in trading volumes and therefore payment/transaction activity.
For financial criminals, this is the ideal opportunity to do their activity in plain sight because there is so much money flow in the market. However, rules-based systems may not be able to detect this as the payment activity appears as legitimate.
Another case is transfer pricing - the legal use of tax laws to minimise tax liabilities for multinational entities. However, to rules-based transaction monitoring systems, the pattern of payments and transfers between accounts could look an awful lot like tax evasion - one of the most common predicate crimes for money laundering - and will therefore be flagged. Legitimate activity is identified as being suspicious - raising yet another false-positive alert that must be investigated. High levels of false positives are endemic in transaction monitoring and contribute to significant operational costs.
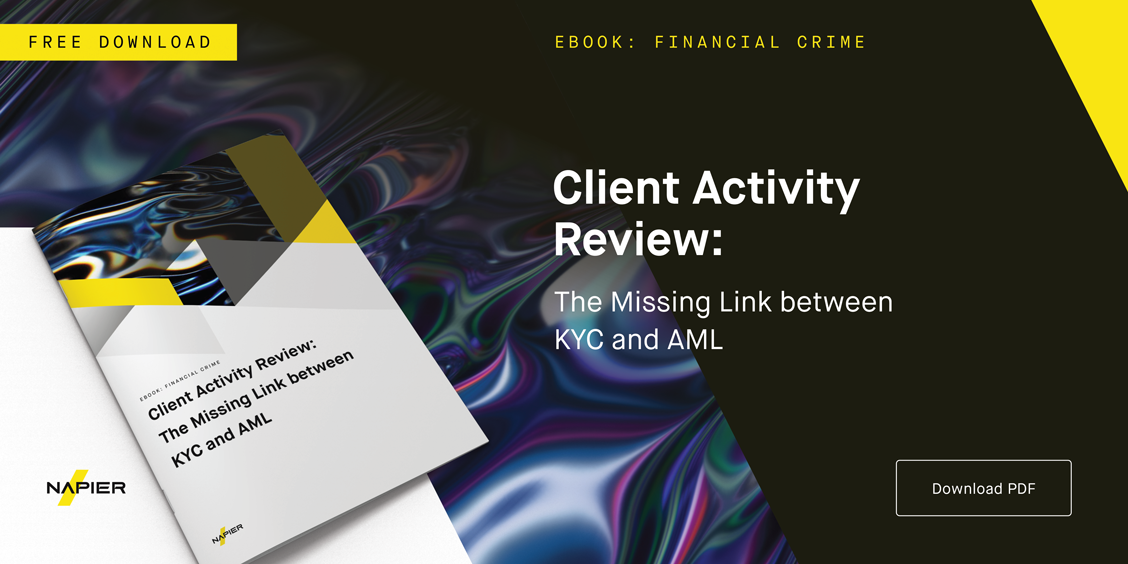
Client Activity Review: The Missing Link Between AML and KYC.
How does customer behaviour analysis work?
Key to customer behaviour analysis is the ability to create customer profiles that can allow comparisons between the current and previous behaviour of that single customer and comparisons to similar customers. The segmentation of similar customers is performed according to several characteristics - for corporates it may be by industry and/or by size and/or geographical location.
Most money-laundering is performed by companies rather than individuals - they have legitimate reasons for transferring money, whether it’s to pay salaries, utility bills, rent etc. By capturing this information, systems such as Napier can build up patterns of expected legitimate behaviour for customers in the same segment. This then enables analysts to answer questions such as for a company of this size, are the monthly payments commensurate with the level of turnover for this company relative to its peers?
The more data that can be captured about a company - what they do, where they do it, who their customers and suppliers are, accounts payable data, credit records etc - the more complex behaviour can be captured and identified. For example, Napier can be trained to look for characteristics in patterns of transactions that indicate transfer pricing as opposed to tax evasion, greatly reducing the number of false positives.
Increasing the sophistication of customer behavioural analytics can bring even more power to the detection of suspicious activity. By their very nature, it can be hard to identify patterns of illicit behaviour relating to criminal enterprises but it is possible to use other similar but legitimate businesses as a proxy.
For example, if we wanted to create a model that could detect patterns of behaviour associated with the illegal trafficking of women we could look at legitimate businesses in some Asian countries that are set up to recruit foreign workers and help them to obtain the correct visas so they can work legitimately as domestic servants or in less regulated parts of the labour such as karaoke bars and massage businesses. In the latter cases, payments are often made using local e-payment services systems allowing data to be obtained to help model networks and associations that can then be applied to identify recruiter businesses that are involved in human exploitation and the illegal trafficking of women to other markets.
This level of sophisticated modelling and analysis requires a lot of data and analytical power and only the largest and well-resourced financial institutions currently have this capability.
How can financial institutions begin to analyse customer behaviour for AML?
Regulated firms can still get benefits from analysing customer behaviour without the need for huge data sets and dedicated research and analytical teams.
The first step for any size organisation is to understand the data that you already have.
● Is your data of good enough quality - in terms of both completeness but also integrity - to give you additional insights?
● What do you need to do to improve the quality and intelligence in the data you already have?
● Can you review all of the suspicious activity reports you have made in the last year and use data analytics to identify patterns?
● Can you begin to integrate data from different sources (such as their customers and suppliers, accounts payable, historical behaviour) to add context to what is known about your customers?
● Can you view your customer and transactional data together?
● Can you begin to segment your customers based on various characteristics?
● Can you establish behavioural causality?
From this, you can identify both risks and opportunities for structuring your data better to gain more intelligence and insights and obtain more accurate and consistent results that are continuously improved over time. Napier’s Client Activity Review is the ideal tool for beginning this process, combining all customer data, including transactions, payments and screening results (such as underlying changes in beneficial ownership and KYC data), into one automated control centre which allows you to triage all anomalies and behavioural changes in light of a full view of the customer.
Bad actors do not sit around and sadly, this has been clearly demonstrated during the COVID-19 pandemic. The huge shift to doing everything digitally has created new opportunities for bad actors to exploit, new ways for them to hide in plain sight. Customer behaviour has also changed significantly, adapting to a different normality. Traditional rules-based systems simply aren’t sophisticated enough to recognise what is suspicious and what isn’t. Now, more than ever is the time to use the data you have about your customers to more effectively identify financial criminality and make the world a safer place.
Looking to understand your customers?
Contact us to speak to one of our experts, or request a demo of our systems to see how we can transform your AML compliance.