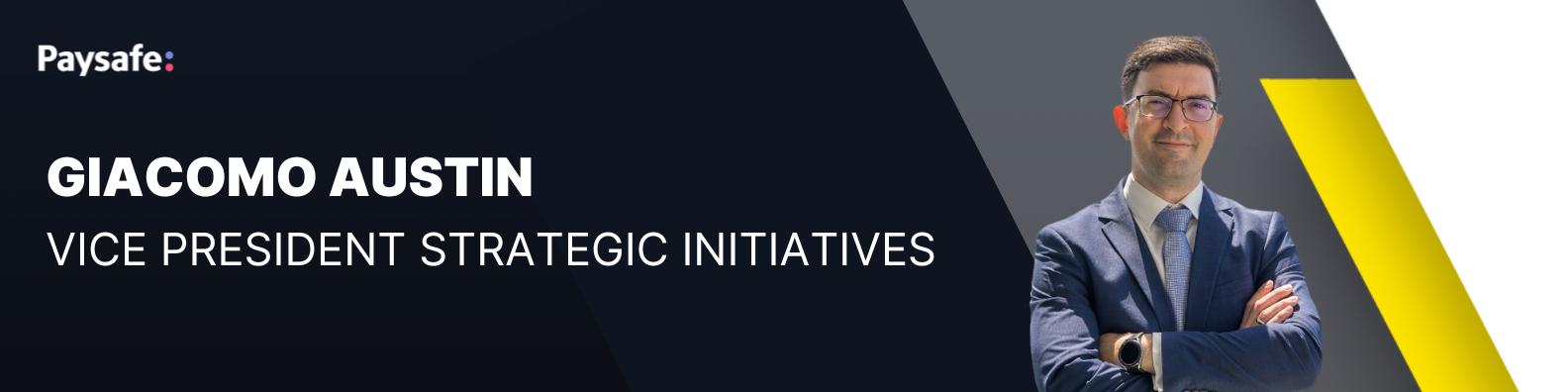
Giacomo is an experienced leader in managing people and driving organisational change, with a diverse background spanning various industries and global contexts. Previously, he led the Compliance team at Paysafe Group, overseeing operations across different time zones and locations and successfully managing significant projects such as mergers and acquisitions and regulatory compliance efforts. In his current role within the Strategy & Transformation unit of the Growth department, Giacomo is responsible for coordinating the execution of the Growth roadmap, and implementing innovative work practices.
Payment providers are currently up against a range of compliance challenges, from the difficulty of keeping up with sophisticated fraudsters during the rise of real-time cross-border payments, to the ever-increasing consumer demand for fast, seamless and safe payment services. The payments industry is continuing to drive towards cheaper and faster payments alongside a more transparent service for consumers. It’s getting harder to stay ahead of the risk curve, especially as across the industry, the push for modernisation is hindered by outdated technology and processes which need to be balanced with the rising need for cost management.
Machine learning has a significant role to play in compliance across all payments providers, whether you’re an acquirer, merchant or issuer and understanding the role it can play in automation and decision making is key to making it work for your organisation.
Deriving data insights
Machine learning can use rules and data sets to derive insights into behaviours and patterns to help build better customer profiles for Know Your Customer (KYC), Anti-Money Laundering (AML) and Anti-Fraud requirements. It can also help to automate other Compliance and Risk-related activities.
Examples of this include:
- Risk assessment and prediction: including fraud detection and risk scoring based on indicative patterns that the algorithms can assign risk scores to.
- Automated monitoring: transaction monitoring (TM) models can automatically monitor in real-time and create suspicious activity reports (SARs) for compliance teams.
- Regulatory reporting: machine learning models can assist in the automated generation of compliance reports, ensuring that all necessary information is accurately compiled and submitted on time.
- Pattern recognition: models can support analysts to identify complex patterns across millions of different data entries, whether that be in customer login data or in the images submitted for KYC.
- Case resolution decisioning: models are ideally suited to learning from analyst case resolutions and apply that to automate the majority of decisioning going forward.
- Training and education: machine learning models can be employed to keep compliance teams updated on the latest regulatory changes, providing personalised training based on individual needs.
- Root cause analysis: machine learning models can assist teams in identifying the root causes of compliance issues by analysing a large volume of data to uncover patterns and correlations.
Automation & decision making
Machine learning is a valuable asset for speeding up decision-making activities, which causes time inefficiencies. It does this by utilising the insights based on your existing rule sets and your historical analyst decisioning. For example, audit trail analysis can be used to log any irregularities or suspicious activities, facilitating timely detection of potential compliance violations and freeing up analyst's time for more complex investigations.
Building trust in machine learning
It’s important to build trust in ML, and this can be achieved through clear and concise information on how the ML models work and what they aim to do.
Explainability is something that we often talk about in our industry, but it really is the foundation on which we build trust not only within our compliance teams but also within other functions and externally with our regulators and other stakeholders. - Giacomo Austin, Paysafe
While regulators are demonstrating more openness towards machine learning models being used in the context of anti-financial crime efforts, this can only work if there is full transparency and explainability for the outcomes and process followed by the models. Black box decisioning is not at all seen in a positive light. Moreover, it is critical that we demonstrate compliance with GDPR and other privacy laws when using customer data and when launching a new model.
Overcoming ML biases
There’s a fear factor around ML and artificial intelligence (AI) running away from us and developing stereotypes and biases in decision making but there are a few ways to overcome this inertia.
- Data cleanliness
Work with your team to ensure good data hygiene as consistently as possible, completely clean data is hard to achieve but it’s something that we can strive for to continue to build trust in ML models.
- Setting and managing your own rules
Rules based logic is based on rules that you and your team set and control, so if there is an issue with the derived insights then look at your data and rules to see what’s going on but remember you’re in control.
- Ensure you have the right variety, diversity, and expertise in your decisioning.
Models that learn from a small silo of analysts who all think the same way are bound to replicate that behavior. Similarly, if most of the decisions feeding into the model are made by very junior analysts then that is the decisioning the model will learn. It is important to ensure different perspectives feed into the models and that suitably expert staff are reviewing those decisions.
Of course, there’s a risk of biases in anything that is learning and that’s why it’s key to be careful about the data you’re feeding you ML models and have authority over how your team are employing it.
For example, biases we often see creeping into machine learning models come from bad data or data points that might be driven by regional data or by incorrect analyst decisioning, so rebalancing your input points is important; remember that something that is a consistent pattern might not be a bias and might be true based on your data and the current risk landscape.
Machine learning in the future
In short, the possibilities of ML within compliance are endless but it requires good data hygiene, community collaboration and investment in the long-term investment of time and resources to truly innovate the way it’s used within compliance (and in many other fields). Remember, machine learning is not here to replace industry experts within compliance but support them with automation and decision-making insights to help us become more efficient as a financial crime fighting community.
Want to streamline and monetise financial crime compliance for your institution? Read our latest eBook:
